When machines take the tasks, humans must own the meaning.
The Ghost of Accountability in the Machine
In the old playbook of management, RACI – Responsible, Accountable, Consulted, Informed – served as a tidy taxonomy for human collaboration. It worked, back when every actor in the system had a mind, a conscience, and skin in the game. But now a new kind of agent has entered the org chart: artificial intelligence.
We’ve awakened a dazzling executor – a machine that can perform tasks, surface insights, and even offer advice. In RACI terms, AI can be Responsible, and it can be Consulted. But here’s the crux: it can’t be Accountable, and it certainly can’t be meaningfully Informed. Accountability demands moral weight and the capacity to answer for outcomes. Being informed requires more than access to data – it requires understanding, context, and relevance. AI has none of these. It can act, but it cannot own.
So RACI, once rock-solid, starts to crack under pressure. One of its core roles – the one that answers for everything – cannot be filled by a non-human agent. The result is something curious: a split definition of AI.
- Artificial Intelligence: the agent that does.
- Accountable & Informed: the human who knows and owns.
This isn’t just clever wordplay. It exposes a deep structural gap in how we delegate authority in the age of automation. The illusion that “the algorithm did it” unravels the moment things go wrong – because no one taught the algorithm how to care.
And that’s where the real trouble starts.
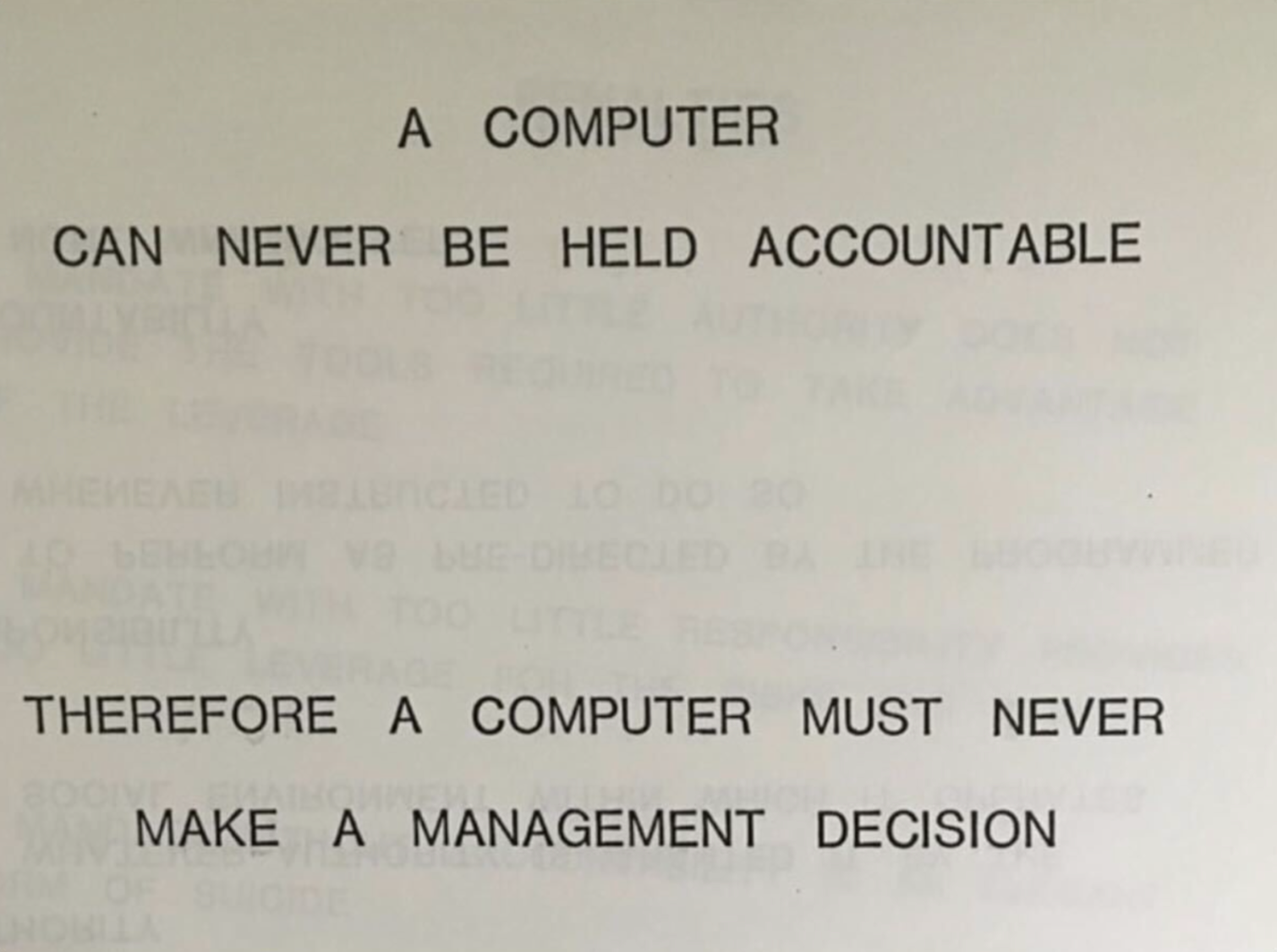
Absurd Consequences of Unattended AI
Handing the car keys of responsibility to an unthinking AI while no one occupies the driver’s seat can lead to outcomes that range from the comedic to the catastrophic. Unattended AI can diligently follow its code into truly absurd territory.
For example, one innocuous Facebook ad showed nothing more risqué than a batch of onions – yet the platform’s automated guardians freaked out. The AI, tasked with zapping nudity, flagged the onions as “overtly sexual” and banned the ad. Facebook later admitted its system “doesn’t know a walla walla onion from a, well, you know” . In other words, the poor bot couldn’t tell a vegetable from a private part. The result was equal parts absurd and telling: without human judgment, the AI’s “responsibility” to filter content turned into a farce requiring human intervention and apology. And this isn’t an isolated stunt – overzealous content moderators have repeatedly taken down perfectly innocent posts (even snippets of historical documents) until a human steps in to set things right .
In the financial realm, the story shifts from whimsical to outright perilous. Think of a trading algorithm as a tireless sorcerer’s apprentice on Wall Street: capable of executing thousands of orders in a blink, but with no wisdom to know when to stop. One famous morning in 2012, a leading firm switched on a new high-frequency trading algorithm…and chaos ensued. The program misfired, buying high and selling low in a frenzied loop, and in just 45 minutes it churned out 4 million unintended stock trades, wildly swinging prices (one stock jumped from $3.50 to $14.76 in minutes) . By the time stunned humans pulled the plug, the algorithm had vaporized $440 million of the company’s wealth . There was no malice, no emotion – just relentless computation following its flawed instructions to absurd extremes. The “responsible” agent (the AI) did exactly what it was told, and in doing so revealed that responsibility without judgment is like a runaway train with no conductor.
In policing and justice, the stakes of unthinking automation are even more fraught. Police departments have tried using predictive algorithms as crystal balls to foretell crime – only to find these oracles can be grossly, statistically wrong and socially biased. One city’s “advanced” crime prediction software was later found to be correct less than 1% of the time , essentially spewing random false alarms. In another notorious case, an AI risk assessment tool incorrectly labeled Black defendants “high-risk” at nearly double the rate of white defendants , reflecting and amplifying societal biases. The outcome? Potentially harsher treatment of individuals because a formula said so. Here the absurdity isn’t humorous – it’s an injustice. An algorithm that can barely grasp context or history was entrusted with decisions impacting real lives. Such examples highlight a sobering truth: when AI operates unsupervised in roles of great consequence, it can produce results that are not just erroneous, but ethically absurd. And still, when the predictive-policing AI fails, it is humans – police officers, judges, communities – who must face the consequences and clean up the mess. The algorithm itself certainly won’t lose any sleep over the people it misjudged.
Counting What Can’t Be Counted
At the heart of these fiascos lies an epistemic tension between quantification and qualitative judgment. AI is, at its core, a master of quantification – it reduces the world to numbers, patterns, weights and probabilities. This can give it superhuman speed and scope in analysis. But what an AI cannot do is qualitatively understand meaning or context the way a human can. It doesn’t truly know why a picture of onions is different from obscenity; it doesn’t comprehend why a sudden 400% jump in a stock price is suspicious; it has no sense of the historical and human context behind crime statistics. It optimizes for what it was told to optimize – clicks, engagement, profit, arrests – all metrics devoid of the rich qualitative tapestry of real life where truth isn’t always numeric and not everything that counts can be counted.
This is a fundamental truth of our human-AI collaboration: AI can calculate, but it takes a human to contemplate. We see the world not just as data points but as narratives, principles, and experiences. Where an algorithm sees correlation, a human sees causation and moral implication. Where the machine tallies figures, the human mind seeks truth – that elusive alignment of facts with reality and justice. We often imbue AI with the aura of objectivity (“the computer says so, therefore it’s true”), yet AI’s outputs only reflect the data it’s given and the goals it’s assigned. It lacks an innate compass for honesty or fairness. As a result, there is a dangerous temptation to abdicate judgment to the algorithm – to trust the quantification over our own qualitative insight. That is precisely when things go wrong. When we treat a statistical proxy as the whole truth, we become untethered from reality. The lesson from these AI slip-ups is that human overseers must stay intimately in the loop, grounding decisions in common sense, context, and ethics, no matter how impressive the numbers look.
Toward an A.C.T.I.V.E. Framework
If the venerable RACI matrix is ill-suited to our AI-centric world, how do we re-imagine roles and responsibilities? Perhaps the answer is to become more A.C.T.I.V.E. – a framework in which humans and AI collaborate, but humans maintain the facets of accountability that algorithms inherently lack. “RACI is dead; long live A.C.T.I.V.E.” might be our new rallying cry. Each letter of A.C.T.I.V.E. can serve as a reminder of the living qualities of leadership and oversight that we must uphold in the age of AI:
A – Anchor of Accountability: However capable an AI may be, a human leader must remain the anchor, ultimately answerable for the results. Just as a ship needs an anchor to stay grounded, organizations need an accountable human to stay moored to reality and consequences. This means owning the outcomes (good or bad) of AI-driven decisions and being prepared to justify or rectify them. True accountability is a weighty anchor that no machine can carry – it requires a conscience and the capacity for remorse, learning, and growth.
C – Compass of Conscience (and Context): AI can crunch data, but conscience is the uniquely human compass that points to right and wrong. We must consult not only algorithms but our moral intuition and contextual understanding. This is about preserving a human-in-the-loop who asks: “Is this action just? Does it align with our values and the broader context?” Rather than a sterile “Consulted” role, C reminds us of our duty to serve as the ethical compass and contextual guide for AI’s raw calculations.
T – Torch of Truth: In a landscape often fogged by dashboards and KPIs, we need a torch to illuminate the truth beyond the metrics. This means actively validating AI’s outputs against reality, seeking the why behind the what. Are we measuring what truly matters, or just what’s easy to quantify? Shining a light on qualitative factors – the stories, anomalies, and lived experiences behind the data – guards against blindly following a false signal. Truth-seeking is an ongoing journey, not a static data point, and it takes human curiosity and skepticism to carry that torch.
I – Insight and Intuition: Information alone is not insight. AI can firehose us with data and analyses, but it’s the human ability to synthesize, interpret, and intuit meaning that turns that into wisdom. This component urges us to go beyond being merely “Informed.” We must cultivate insight – the deep understanding that comes from mixing data with experience, intuition, and imagination. Sometimes our gut feeling or anecdotal observation might override an AI’s recommendation, and rightly so. An active partnership with AI means valuing our own intuitive insights as a complement to algorithmic outputs.
V – Values and Vision: Where do we ultimately want to go, and what principles do we refuse to compromise along the way? AI has no inherent values; it will optimize whatever we ask it to, even if that leads off a cliff ethically. Human values – fairness, compassion, integrity – must therefore frame every AI deployment. This “V” reminds us to imbue our strategies with a clear vision of human well-being. It’s about actively checking that AI’s actions align with our core values and long-term vision, rather than undermining them. In short, never outsource your moral compass.
E – Ethical Discernment: Finally, even with all the above, situations will arise that demand nuanced judgment calls – ethical dilemmas, unprecedented scenarios, the gray areas where rules don’t neatly apply. Ethical discernment is the art of deliberation, weighing the lesser of evils, and consulting our shared humanity in decision-making. This is a distinctly human realm. Being ACTIVE means reserving the final say for human ethical review. It’s ensuring that empathy and prudence prevail over cold logic when it really counts. We must be the ethical failsafe, ready to intervene when the letter of an algorithm’s law violates the spirit of justice.
By reframing RACI into this more evocative A.C.T.I.V.E. mindset, we acknowledge that while AI is an incredibly powerful tool – a dutiful apprentice – the master of the process must remain actively engaged. It’s not enough to assign roles and walk away; we have to live those roles in an ongoing, mindful way.
The Human Edge in the Age of AI
Full accountability in the age of AI involves a deep and dynamic relationship with truth, grounding, and ethical discernment. It means recognizing what only humans can do: imbue cold data with warm wisdom. AI will continue to assume more responsibilities in our organizations – and rightly so, for its capabilities are astonishing – but we should never be lulled into thinking we can automate accountability itself. The soul of accountability lies in understanding, compassion, and truth-facing honesty. Those qualities can’t be coded into a machine.
So, yes, RACI is dead in the sense that we can no longer tick the “Accountable” box next to an AI’s name and call it a day. The new world demands that we be more A.C.T.I.V.E. than ever. We must step forward as philosophical captains, holding the wheel firmly even as our automated crew tirelessly rows. Only by doing so can we harness the immense potential of AI without losing our own north star – our humanity – in the process. In this dance of man and machine, it is ultimately up to us, the humans, to lead with conscience and courage. Accountability isn’t a role that can be coded; it’s a relationship with reality and truth that we must each actively uphold. RACI is dead; long live active accountability.