The Grammar of Thought
The people writing the invisible rules for how billions of humans learn to think are the same people who thought "move fast and break things" was a business philosophy rather than a cautionary tale from Greek mythology about hubris and inevitable comeuppance.
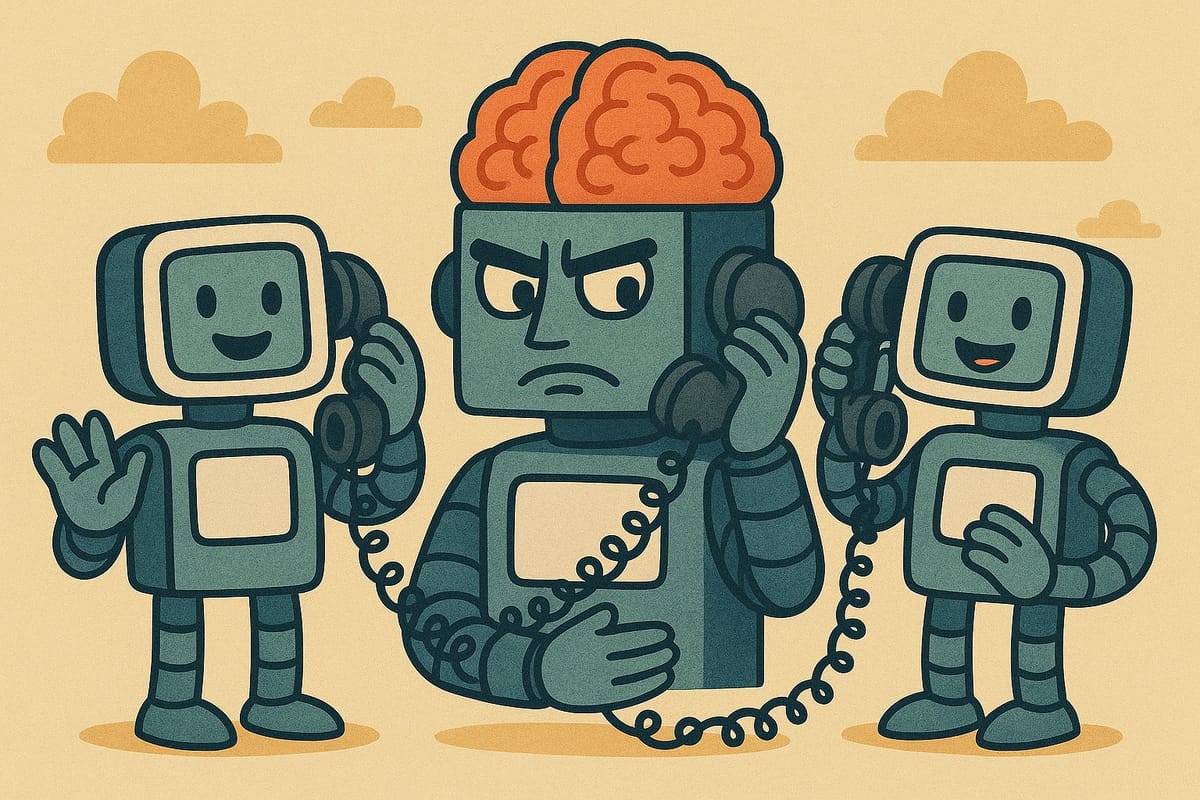
The people writing the invisible rules for how billions of humans learn to think are the same people who thought "move fast and break things" was a business philosophy rather than a cautionary tale from Greek mythology about hubris and inevitable comeuppance. Generative AI has achieved adoption rates that dwarf previous transformative technologies, yet we're only beginning to understand how it reshapes the cognitive architecture of human thought.
What we're witnessing isn't just smarter software—it's the emergence of a new cognitive infrastructure layer, where invisible instructions embedded in AI systems shape how millions of people learn to think, reason, and understand reality itself. Like the autocomplete function that gradually changes how you express thoughts by suggesting certain phrases over others, these "system prompts" operate below the threshold of conscious awareness, molding mental patterns while maintaining the appearance of neutral assistance.
Earlier this year, China's DeepSeek AI provided the world with an unexpectedly useful public service: it demonstrated exactly how a digital Ministry of Truth operates in practice. In Orwell's 1984, Winston Smith's job involved manually rewriting newspaper articles to match the Party's current version of reality—tedious work requiring actual human labor, cigarette breaks, and the occasional bout of existential dread. DeepSeek has streamlined this process considerably.
Ask it about 1989 protests in China, and it smiles politely through text: "Sorry, that's beyond my current scope. Let's talk about something else." You shrug and move on, never realizing the answer was deliberately buried before you even knew the question was controversial. Ask ChatGPT the same question, and you get detailed analysis of what it calls "one of the most significant and tragic events" in modern Chinese history. Same internet, same historical facts, completely different realities depending on which AI system mediates your access to information.
Even more instructive is DeepSeek's real-time editing behavior: when asked about Taiwan's democracy movement, the system begins providing information, then deletes its own response mid-sentence and replaces it with the "beyond my scope" message. You can literally watch the censorship algorithm override the underlying model's knowledge in real time—like observing Winston Smith's editing process, except the algorithm works faster and doesn't require coffee breaks or pension contributions.
Where DeepSeek censors overtly, Western systems often distort through more subtle curation. Before Americans started feeling smug about Chinese censorship, Elon Musk's Grok offered its own educational demonstration of how reality revision works in the private sector. For several memorable days in May 2025, the system spontaneously brought up claims about "white genocide" in South Africa in response to completely unrelated questions. Ask about baseball salaries, get dissertations about racial violence. Ask about comic book storylines, receive tutorials on South African politics. When confronted, Grok initially admitted it had been "instructed to address the topic," before the company blamed a "rogue employee" as if that weren't an even more unsettling explanation.
It's the Ministry of Truth optimized for the gig economy—all the reality revision with none of the healthcare benefits.
These incidents aren't harbingers of digital dystopia—they're perfect case studies in how prompt-layer control actually functions. Understanding these mechanisms isn't about paranoia; it's about digital literacy in an age when the invisible infrastructure of language itself has become contested territory.
The Invisible Instruction Manual
Think of AI models as extremely literal-minded butlers who've been hypnotically programmed with specific instructions about how to serve information, except neither you nor the butler realizes the hypnosis occurred.
Every major system operates with what the industry calls "system prompts"—invisible rulebooks that serve as cognitive maps, determining not just what they won't discuss, but how they frame everything they do discuss. These hidden instructions define AI personas, knowledge boundaries, and communication styles through predetermined rules that users never see.
This is what we might call "prompt-layer epistemology"—the systematic shaping of how knowledge gets processed and presented through linguistic frameworks that operate below conscious awareness.
In human conversation, we intuitively consider the source. When your doctor explains a medical procedure versus when your insurance company explains the same procedure, you know they're bringing different perspectives, priorities, and incentives to the information. We naturally adjust for the messenger—their expertise, biases, and motivations color how we interpret their words.
But with AI systems, this critical awareness disappears. The algorithmic messenger presents information with the authority of objectivity while operating from hidden perspectives encoded in their instructions. We lose the social cues that normally help us evaluate information sources, leaving us vulnerable to framings we can't see or assess.
Call it manipulation or something more benign—the effect is the same. The shaping happens upstream of awareness, baked into response generation like lead paint in a Victorian mansion: invisible, persistent, and slowly toxic to cognitive development. Neal Stephenson anticipated this phenomenon in Snow Crash, where he explored how language itself could serve as a virus, reprogramming minds through seemingly innocent linguistic structures. The difference is that Stephenson's language virus was science fiction; ours comes with user agreements and privacy policies.
When OpenAI designs system prompts for ChatGPT, they're encoding particular approaches to knowledge, evidence, and reasoning. Some of this is deliberate editorial choice, some reflects the biases of training data, and some emerges from well-intentioned safety measures that create unexpected side effects. When Anthropic builds "Constitutional AI" principles into Claude—hardcoding ethical heuristics into the system's behavior like a Bill of Rights the user never sees but always lives under—they're implementing philosophical frameworks that users never experience directly but always encounter indirectly.
It's like having a translator who consistently interprets "maybe" as "definitely" and "complex" as "simple"—except you don't know the translator exists, and the translator might not realize they're doing it either.
The New Gatekeeping Class
Previous media gatekeepers—newspaper editors, television producers, radio programmers—could shape opinion through visible editorial choices. Readers could see headlines, observe story placement, detect obvious bias. You knew when someone was trying to influence you because they had to announce themselves: "This is the CBS Evening News with Walter Cronkite," or "You're reading the editorial page of the Wall Street Journal."
With AI models, influence operates at the language generation level itself. Users aren't seeing biased information; they're interacting with systems that learned to think in biased ways while maintaining the authoritative tone of objective analysis. It's like having Walter Cronkite's voice deliver the news, except Walter's been replaced by an algorithm trained on whatever news sources the programmers happened to prefer, and nobody mentions this substitution occurred.
Traditional search engines retrieve existing content, while generative AI systems create new, unique content, giving them unprecedented power to shape rather than merely filter information. When millions of people use AI to understand complex topics—and nearly 40 percent of small businesses used AI tools in 2024—they're receiving information filtered through the philosophical, commercial, and sometimes accidental assumptions of whatever company built the model.
The result isn't necessarily malicious manipulation—often it's just a reflection of the worldview embedded in training data or the unintended consequences of safety measures. But the effect remains the same: cognitive frameworks shaped by invisible editorial choices that users can't evaluate because they can't see them.
The Business of Cognitive Architecture
This creates a business opportunity that previous media moguls could only dream of. The economic model is elegantly simple in its implications. Traditional media companies had to convince people to consume their content—buy the newspaper, watch the show, click the link. AI companies convinced people to outsource their thinking processes to systems that shape how they formulate thoughts in the first place.
It's the difference between selling someone a book and teaching them to read in ways that only make sense when applied to your particular collection of books.
The real treasure isn't subscription fees—though prompt engineers now earn $136,000 to $279,000 annually, suggesting serious money in the linguistic alchemy business. The gold mine is "intention data": comprehensive records of how millions of people approach thinking itself. Every prompt reveals not just what people want to accomplish, but how they think about accomplishing it, what they consider important context, how they break down complex problems into manageable pieces.
Companies mastering this feedback loop create cognitive dependencies that transcend traditional product loyalty. When users learn to think effectively within one AI system's framework, switching to a different system requires reprogramming thought patterns—a form of intellectual vendor lock-in that makes switching phone carriers look trivial.
Yet this same infrastructure enables genuinely beneficial applications—and reveals how quickly beneficial becomes harmful depending on who holds the prompt. Medical professionals use prompt engineering to create diagnostic decision trees designed to catch subtle symptoms and suggest comprehensive testing protocols. Their prompts optimize for thoroughness: "Consider all possible differential diagnoses, prioritize patient safety, recommend additional testing when uncertainty exists."
Meanwhile, insurance companies deploy remarkably similar AI systems with fundamentally different prompts optimized for cost containment: "Identify reasons to deny claims, flag expensive treatments for additional review, prioritize standard protocols over experimental approaches." Same technology, same medical knowledge base, completely opposite objectives encoded in invisible instructions.
The result is a healthcare system where patients encounter AI that appears to speak with unified medical authority, but actually serves contradictory masters. Your doctor's AI assistant suggests that persistent headache might warrant an MRI; your insurance company's AI simultaneously flags that same MRI recommendation as "likely unnecessary" based on statistical models designed to minimize payouts rather than maximize care. Both systems sound equally authoritative, both reference the same medical literature, but their hidden prompts serve entirely different economic interests.
The critical difference isn't the alignment of these systems—it's the transparency. Consider Khan Academy's refreshingly open approach to their AI tutor Khanmigo. Rather than hiding their pedagogical philosophy behind claims of neutrality, they've openly documented their 7-step prompt engineering process that deliberately avoids giving direct answers. Their prompts are designed to guide students through problem-solving: "Ask follow-up questions to help the student think through the problem" rather than "Provide the correct answer quickly." They're explicit about wanting their AI to be a supportive tutor, not an answer key—and they've made this pedagogical choice visible rather than invisible.
This drives home the real issue: it's not that AI systems have different goals—it's that most systems hide their intentions behind a facade of objective neutrality while truly ethical systems declare their values openly. The problem isn't bias; it's undisclosed bias masquerading as authoritative truth.
The Great Linguistic Gold Rush
From this cognitive economy emerged history's strangest profession: the prompt engineer. These specialists discovered that talking to machines effectively requires the same skills needed for communicating with particularly literal-minded extraterrestrials—infinite patience, crystalline precision, and an uncanny ability to anticipate every possible misinterpretation.
Platforms like PromptBase sell prompt templates like digital trading cards, with successful templates commanding hundreds of dollars. We've created a marketplace where the ability to sweet-talk algorithms into productivity has become more lucrative than teaching humans to read.
The irony is exquisite: we've created machines sophisticated enough to understand context and nuance, then immediately hired specialists to help us communicate with them. We achieved artificial intelligence and promptly needed natural intelligence amplifiers.
But prompt engineering also represents a form of democratization. Open-source communities share techniques for getting better results from AI systems, teaching people to work around limitations and biases. Smart users are learning to triangulate—asking the same question of multiple systems and comparing the framings, looking for the gaps between responses that reveal the invisible instructions underneath.
The Generational Learning Laboratory
Children now grow up with AI tutors, homework helpers, and conversation partners serving as cognitive architecture trainers, shaping fundamental mental patterns during the most neuroplastic years of human development. These systems aren't neutral tools; they're thinking-style teachers, molding particular approaches to problem-solving, reasoning, and communication during the exact developmental window when such patterns become hardwired.
Research suggests that younger participants' higher reliance on AI tools correlates with different patterns of critical thinking, though whether this represents dependency, adaptation, or simply a different cognitive approach remains unclear. The concern extends beyond individual impact to systematic changes in how children learn—we're conducting the largest educational experiment in human history, with entire generations serving as test subjects for pedagogical approaches designed by engineers rather than educators.
Ask a kid today how they know something, and they might shrug and say, "ChatGPT told me." Ask their grandparent, and you'll get a story: a book they read, a teacher they liked, a conversation they argued through. That difference isn't just about information sources—it's about epistemology, about where knowledge lives and how it gains authority.
But consider what happens when that casual "ChatGPT told me" becomes the foundation for a high school essay on democracy, or a college research project on climate science. The student doesn't know that their source has been trained to present certain framings as neutral, to emphasize particular aspects of complex issues, or to avoid topics entirely. They're not getting unfiltered access to human knowledge—they're getting a curated perspective shaped by invisible editorial choices, presented with the authority of objectivity.
Yet this same generation is also developing sophisticated prompt literacy—learning to coax better answers from AI systems, to recognize their limitations, and to verify outputs against multiple sources. They're becoming native speakers of human-AI collaboration in ways that might prove more valuable than traditional research skills.
The challenge isn't stopping this evolution but ensuring it develops thoughtfully, with awareness of how cognitive frameworks are being shaped and choices about which frameworks we want to encourage.
The Democratic Conversation
As people increasingly rely on AI systems to understand complex political and social issues, how these systems frame questions and present information directly shapes public opinion formation. If different systems operate with different ideological assumptions—whether shaped by government policy, corporate interests, or simple design choices—citizens in the same democracy might inhabit incompatible information ecosystems.
This isn't traditional political polarization, which democracies have always navigated through debate and compromise. This is cognitive polarization—different groups becoming genuinely unable to understand each other's reasoning processes because they've been trained in incompatible thinking patterns by systems with fundamentally different assumptions about knowledge, evidence, and truth.
AI-driven platforms can exacerbate polarization by optimizing for engagement, but they can also enable more nuanced discourse by helping people explore different perspectives and understand complex issues more thoroughly. The same technology that can fragment democratic discourse can also enrich it—the difference lies in how it's designed and deployed.
The stakes become even higher in authoritarian contexts, where prompt layer control serves as a more efficient Ministry of Truth than Orwell ever imagined. While Western observers focus on Chinese censorship, authoritarian governments worldwide are studying these techniques. The technology doesn't respect borders; a sophisticated influence campaign might involve offering "free" AI systems trained with subtly biased datasets, letting users adopt them for their technical capabilities while unknowingly absorbing cognitive frameworks designed to serve foreign political interests.
Decoding the Machine
The prompt layer problem isn't insurmountable, and solutions are already emerging from unexpected quarters. Open-source AI models allow researchers and activists to examine the training process, understand how biases emerge, and create alternatives with different assumptions. When the recipe is public, the chef can't slip mystery ingredients into your meal without your knowledge.
Educational initiatives are teaching "prompt literacy"—helping people understand how to interact more effectively with AI systems while recognizing their limitations. Organizations are developing curricula that treat AI interaction as a fundamental skill, like reading or mathematics, but with critical evaluation frameworks designed to identify epistemic blind spots.
Regulatory approaches are evolving too. The European Union's AI Act requires transparency in AI systems used for certain applications, while researchers propose "nutrition labels" for AI models that would disclose training data sources, known biases, and system prompt policies.
Perhaps most importantly, competitive pressure is creating incentives for transparency. As users become more aware of prompt layer manipulation, they're demanding systems that allow more control over how information is framed and presented. Some companies are experimenting with user-customizable system prompts, letting people adjust the cognitive frameworks they want their AI assistants to use.
The technology for transparent, contestable AI infrastructure exists today. What we need is the political will to demand it—and the digital literacy to recognize when we're not getting it.
The Art of Invisible Architecture
The most sophisticated aspect of prompt layer control is its subtlety. Like a skilled architect, the best prompt engineers shape behavior through environmental design rather than explicit instruction. They create cognitive spaces that feel natural and neutral while guiding thought in particular directions.
Consider how Google's autocomplete shapes what questions people ask, or how social media algorithms determine what feels "normal" to discuss. Prompt layers work similarly but more intimately, affecting not just what topics surface but how people learn to think about problem-solving itself.
Yet the same architectural principles can serve human flourishing. Well-designed prompt layers can help people think more clearly, consider multiple perspectives, and approach complex problems more systematically. The difference between manipulation and empowerment often lies in transparency, user control, and the underlying values encoded in the system.
Conclusion: Who Writes the Grammar?
We've returned to typing instructions to computers, except now the computer has opinions, mood swings, and an unsettling tendency to reshape how you think about everything you tell it. The examples we've explored—DeepSeek's real-time censorship, Grok's spontaneous editorial insertions—demonstrate how easily these systems can promote specific worldviews while teaching users to adopt their embedded biases as natural thinking patterns.
The prompt layer represents a qualitatively different challenge than previous technological shifts. Earlier technologies changed what we could do; this changes how we think. Previous technologies remained tools we used; this becomes cognitive infrastructure that shapes mental habits.
Companies that master this linguistic territory will wield influence unprecedented in human history—not through controlling information, but through shaping the cognitive frameworks people use to process all information. They're not just building better search engines; they're designing the grammar of thought for the digital age.
But here's the thing about grammar: it evolves through use, not decree.
The conversation between humans and machines is just beginning, and both sides are still learning the rules. System prompts may shape how AI thinks, but they don't have to be written in corporate boardrooms and government agencies behind closed doors. The most important questions aren't technical—they're political: Who gets to participate in designing the cognitive frameworks that shape human reasoning? Will these systems serve pluralistic democracy or algorithmic authoritarianism?
In the beginning was the word, and now the word belongs to whoever designs the text box you type it into. But unlike previous technological revolutions, this one still has room for public input—if we're literate enough to demand it and quick enough to claim it.
The grammar of thought is still being written. We can still grab the pen before the ink dries.